User:HMaloigne/sandbox/Approaches to Knowledge/2020-21/Seminar group 17/Evidence
Week 3: Evidence[edit | edit source]
Quantitive evidence in switching from the Rutherford model to Bohr’s[edit | edit source]
Rutherford's model Immediately preceding Bohr’s atomic model was the Rutherford model, which serves a fine example of the dangers of cherry-picking evidence. Rutherford had done research in atomic radiation, and came to an atomic model with a heavy core of protons and neutrons. This core was surrounded by a cloud of electrons, randomly distributed in a sphere. The model supported new evidence found by Geiger and Marsden in scatter theory, but it failed to explain important chemical questions as valency and the stability of atoms.[1]

Bohr's model With these unanswered questions, there was a clear need of an improved model of the atom. Niels Bohr, a Danish physicist, improved the model of Ernest Rutherford. Bohr based his model on the Rydberg formula that fitted all the observed wavelengths of the hydrogen atom emission spectrum into one formula:[2]
In this equation, fixed values n are used to describe energy levels. He explained this in his model by postulating that the nucleus must be surrounded by electrons in specific orbits with fixed “quantised” energies. In order to prove his theory, the numerical predictions that came from his models had to correspond to experimental data.[2]
And so, evidence was of great importance in the development of Bohr’s module. In the first instance, it was the lack of convincing evidence for Rutherford’s that caused the switch.[1] Additionally, new insight in quantum physics from Max Planck and his Planck constant were important for understanding the concept of quantised energy.[3] And lastly in proving the model in itself: the fact that calculated results Bohr’s model and formula corresponded with experimental data was important in turning his three postulates into a quantitive model.[2]
Evidence in Geography: Defining Water Scarcity[edit | edit source]
Having enough accessible freshwater is vital for socio-economical growth, human health and environmental stability. Freshwater availability is also directly linked with food security. Water scarcity is when there isn't sufficient accessible freshwater to maintain these factors. In order to identify and address water scarcity, different metrics were introduced which shall provide a quantified definition.[4]
The two most commonly used metrics are:
1. The Water Stress Index (WSI):
It measures the number of people using one flow unit of water, defined as 1million m³ per year. The average water demand in a developed country is defined as 100-500 people per flow unit. Water scarcity is set at 1000 people per flow unit.[5]
2. The Withdrawal to Availability Ratio (WTA):
This ratio is as well based on a fixed demand, divided by the amount of freshwater resources available. A WTA ratio bigger than 0.4 indicates water scarcity.
Both these metrics are based on the mean annual river runoff (MARR), which measures the amount of available freshwater by taking the annual amount of precipitation and evapotranspiration into account.[6]
Issues[edit | edit source]
Being simple and universal, there are yet significant disadvantages of these metrics. They fail to cover influential aspects, such as the temporal irregularity of freshwater resources, the societal adaptability, the available groundwater and the moisture saved in vegetation, which often determines the need of freshwater for irrigated agriculture. Not considering the change in spatial distribution of freshwater further prevents society's preparedness for climate change, together with a degree of foreseeable misinterpretation.[6] Additionally, instead of taking the variability of freshwater demand into account, a fixed quantity is used, which is derived from subjective and often oversimplified quantifications of socio-economic factors.[4]
Suggestions[edit | edit source]
Suggestions were made to address these issues: to recognise that the presence of water doesn't equal the accessibility to safe water; to include water storage in the measurement of water scarcity, as it is a way to balance out the temporal freshwater variability; to minimise the subjective quantifications but rather integrate them as physical descriptions of water scarcity into governmental discussions, together with local representatives.[4]
Quantitive evidence and its validity in Epidemiology[edit | edit source]
There are multiple study methods in Epidemiology however these can be divided into two larger categories: either Observational Study Designs or Interventional Study Designs, whereby the researchers are only able to observe existing conditions / lifestyles or can intervene minimally.
Because both Observational and Interventional studies take place in largely non-manipulated circumstances, this can attribute for the factors of bias, confounding, and chance affect the validity of the study's findings.[7]
- Bias: Main two categories of bias are information or selection bias. Information bias occurs when the method of study creates errors in the measuring of a variable, whereas selection bias occurs when participants due to a given set of unaccounted characteristics (for example, they study at the same university).[8]
- Confounding: Confound bias occurs when extra, potentially invaluable, factors are taken into consideration when studying the relationship between an exposure and an outcome, and if these extra factors are then considered a variable affecting the outcome.[9]
- Chance: Chance factors can occur and affect results because a given study will always be limited to its participant group and the time and length of the study, and this chance factor could deviate the result from truth.[10]
Potential Influences of Conclusions in Epidemiology[edit | edit source]
Given the above potential influences on the validity of epidemiological evidence, epidemiologists have set rules to design and account for the potential invalidity of their research. A study must include analyses of its method as well as its findings, outlining all the potential confounds, biases, and possible chance factors that could influence the research outcome, and describing the potential size of this influence. The researchers must also analyse their findings using formal statistics, whereby they can check the precision of their findings based on larger statistics.[11]
Epidemiological research can and does have a lasting affect on the larger societal understanding of health, on national or international policies in health and medical practice, and in pharmaceutical practice and production. These are only some of the reasons for the importance of accounting for the validity of research findings in health-related disciplines.[11]
An example of Bias in Research Findings[edit | edit source]
One 2002 study, the Women's Health Initiative Study, observed a relationship between long-term use of Hormone Replacement Therapy (HRT) and various noncommunicable diseases, and this study shifted the mentality of many health practitioners away from HRT, even though prior to the study over 50 other Observational studies has observed no such relationship.[12]
The problem of evidence distorted by the lack of data on women in medical research[edit | edit source]
Medical knowledge is issued by both theories and experiences. These two manners of obtaining facts are often considered as primary evidence. However, these qualitative and quantitative researches can be biased by a number of factors such as the neglecting of the sex and gender of the subjects used in the study and those it aims to heal.
Women are less present in medical studies. For instance, even though 55% of HIV-positive adults are women, they represent only 19.2% of participants in antiretroviral studies, 38.1% in vaccination studies and 11.1% in studies focused on finding a cure [13] Indeed, it is generally accepted that male and female body do not have to be considered different except for the study of matters related to reproductive function, size and weight.[14] This explains the little number of gender-specific health care research and results.[15] However, it was proven that gender differences also occur at the cell scale, as demonstrated by studies about blood-serum bio-markers for autism and proteins for example.[16] This difference have consequences at a macroscopic scale, for instance for HIV-positive women the symptoms and complications are different than those suffered by HIV-positive men. Hence, medical research should either take the sex of the studied subject into consideration or justify the ignorance of this factor. This common evidence mistake leads to errors and medical misjudgment. It results in ineffective drugs for women and consequently a loss of money.[16]
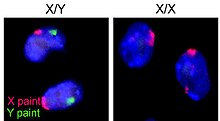
The medical corps justifies this inequality in women data by the difficulty to find available women for tests, the use of hormonal contraception which might impact the results of the research, the lower finding for women research such as the research for coronary artery disease.[16][17]
This evidence problem has been addressed by the NIH Guidelines on the inclusion of women and minorities as subjects in clinical research in 1994.[18]
Use of Quantitative Evidence in Sociology[edit | edit source]
Quantitative evidence is gathered from quantitative research methods, using numerical or statistical data using surveys, surveillance or from administrative records. Quantitative evidence is used to test deductive hypotheses and are widely used in positivist research.[19] Positivism in sociology believes that knowledge in the social world is objective and links between how society and its individuals can be studied like classically scientific disciplines. Using quantitative evidence, positivist researchers aim to find causations and correlations between two social variables and how they impact each other. Quantitative methods provide statistical evidences in the form of numeric figures which could be represented using graphs, charts and numbers.[20]
There are many reasons why positivist sociologists use quantitative evidence in sociology. Firstly, it is very easy to use in hypothesis testing as it directly shows if there are any causations or correlations between variables. Also, most of the methods produce unbiased data which is representative of the population. This is because of the easy to carry out methods which make it easy to test on a larger sample. Apart from the objectivity, quantitative evidence also has a high reliability as the methods could be carried out multiple times to test the hypothesis due to ease in replicability.[21]
Despite this, many sociologists criticise this approach and the use of evidence. This is because it may fail to recognise the differences between the social world and the natural world. Similarly, using methods and procedures may question its ecological validity by presenting a false reality as in most of the methods, external, real-life variables do not affect the evidence.[20] This brings up the question that is quantitative evidence actually capable of producing a reflection of social reality?
Emile Durkheim and the use of Quantitative Evidence in the study of Suicide.[edit | edit source]

A key example of the issues of using quantitative data in studying sociology is Emile Durkheim’s study of Suicide. Despite being a celebrated monograph in the field of positivist structuralism, Durkheim’s study has faced a lot of critique over the years. Durkheim wanted to show how suicide is caused by external social factors rather than individual trouble by linking it to the importance of social integration and a sense of belonging. Suicide statistics of different genders, social class, religions and marital statuses were used to identify what social factors lead to higher rates in suicide. Over the years, this method could be seen as problematic as Durkheim has been accused of committing an ecological fallacy. This is when inferences of the individuals behaviour is inferred from the behaviour as a group, explaining individual actions as a part of society's action.Similarly, the way some groups and statistical records define deaths and suicides also differ (e.g in Catholicism and Protestant Christianity).[22]
Evidence in Complex numbers[edit | edit source]
A complex number is a number of the form , in which and are real numbers, and is the imaginary unit, such that . Because no real number satisfies this equation, is called an imaginary number. [23] The term "imaginary" for these quantities was coined by René Descartes in 1637, although he struggled to stress their imaginary nature[24]
[...] sometimes only imaginary, that is one can imagine as many as I said in each equation, but sometimes there exists no quantity that matches that which we imagine. [...]

These numbers were born out of an attempt to solve quadratic equations that had no real solutions. It was Gerolamo Cardano, an Italian mathematician, who first managed to conceive complex numbers that were the contrary of the existing numbers. [25]In spite of his discovery, mathematicians were still asking questions about the rules governing the imaginary numbers. The way they tried to answer this question was to use previously known and proven properties of Cartesian mathematics and algebra. Operations on complex numbers were then introduced by using extant rules of algebraic operations and relations. Subsequently, the Cartesian plane properties that were well known to mathematicians were utilised to acquaint the Argand diagram. It refers to a geometric plot of complex numbers using the x-axis as the real axis and y-axis as the imaginary axis.[26] Mathematicians used existing evidence to introduce new notions and theorems. That is how most of discoveries in Mathematics were made. René Descartes stated that:
Each problem that I solved became a rule, which served afterwards to solve other problems.
It is such field of study that even if a concept seems to be unfamiliar scholars manage to support and justify it with existing evidence. It is also vital to notice that in this discipline the lack of proof and evidence in a theorem propels the reference to existing proofs.
References[edit | edit source]
- ↑ a b Kragh H. Niels Bohr and the quantum atom. Oxford: Oxford University Press; 2012 [cited 23 October 2020]. Available from: https://books.google.nl/books?hl=en&lr=&id=pVyrkndSrkQC&oi=fnd&pg=PP1&dq=history+of+bohrs+model&ots=dVBqj1soqB&sig=JY0s9CNYWOdzEz5_h6XavVst3Zk&redir_esc=y#v=onepage&q=history%20of%20bohrs%20model&f=false
- ↑ a b c Price S. SDP Lecture 3 [unpublished lecture notes]. CHEM0005: Chemical foundations, University College London; lecture given 19 Oct 20.
- ↑ Bohr Atomic Model [Internet]. Abyss.uoregon.edu. 2020 [cited 22 October 2020]. Available from: http://abyss.uoregon.edu/~js/glossary/bohr_atom.html
- ↑ a b c Taylor, R.G. Rethinking water scarcity: role of storage. EOS, Transactions, American Geophysical Union. 2009;Vol. 90(28): 237-238
- ↑ Falkenmark, M. The massive water scarcity now threatening Africa-why isn’t it being addressed? Ambio. 1989;Vol. 18:112–118
- ↑ a b Damkjaer, S. and Taylor, R.G. The measurement of water scarcity: defining a meaningful indicator. Ambio. 2017;Vol. 46: 513-531
- ↑ JH Zaccai. “How to Assess Epidemiological Studies.” Postgraduate Medical Journal, 2004, 80:140-147.
- ↑ Sacket, DL. “Bias in Analytic Research.” Journal of Chronic Diseases 32, no. 1–2 (1979): 51–63.
- ↑ Last, JM. “A Dictionary of Epidemiology.” Oxford: Oxford University Press, 2001.
- ↑ Wang, Jin Jin, and John Attia. “Study Designs in Epidemiology and Levels of Evidence.” Elsevier Inc., no. 3 (August 5, 2009).
- ↑ a b Rothman KJ, Greenland S. Modern Epidemiology. 2nd Ed. Philadelphia, Lippincott-Raven, 1998. 2nd ed. Philadelphia: Lippincott-Raven, 1998.
- ↑ Rossouw, JE, GL Anderson, and RL Prentice. “Risks and Benefits of Estrogen plus Progestin in Healthy Postmenopausal Women: Principal Results from the Women’s Health Initiative Randomized Controlled Trial.” JAMA, 2002, 288: 321-333
- ↑ Caroline Criado Perez. Invisible Women. Pengouin Random House. UK: Vintage; 2020/ Chapter 10: The Drug's Don't Work; p195-216.
- ↑ Anita Holdcroft, 2007. Available from: https://www.ncbi.nlm.nih.gov/pmc/articles/PMC1761670/#ref6
- ↑ Vera Regitz-Zagrosek1, 2012. Sex and gender differences in health. Science & Society Series on Sex and Science. Available from https://www.ncbi.nlm.nih.gov/pmc/articles/PMC3388783/
- ↑ a b c Caroline Criado Perez. Invisible Women. Pengouin Random House. UK: Vintage; 2020/ Chapter 10: The Drug's Don't Work; p195-216.
- ↑ Anita Holdcroft, 2007. Available from: https://www.ncbi.nlm.nih.gov/pmc/articles/PMC1761670/#ref6
- ↑ Dr. Paulette Gray, Dr. Lore Anne McNicol, Ms. Sharry Palagi, Dr. Miriam Kelty, Dr. Eleanor Hanna, Dr. John McGowan. et al. NIH Policy and Guidelines on The Inclusion of Women and Minorities as Subjects in Clinical Research. Available from https://grants.gov/policy/inclusion/women-and-minorities/guidelines.htm
- ↑ 1. Define Quantitative and Qualitative Evidence | SGBA e-Learning Resource [Internet]. Sgba-resource.ca. 2020 [cited 2 November 2020]. Available from: http://sgba-resource.ca/en/process/module-8-evidence/define-quantitative-and-qualitative-evidence/
- ↑ a b 2. Bryman A. Social Research Methods. 4th ed. New York: Oxford University Press; 2012.
- ↑ Positivism [Internet]. obo. 2020 [cited 2 November 2020]. Available from: https://www.oxfordbibliographies.com/view/document/obo-9780199756384/obo-9780199756384-0142.xml
- ↑ Êmile Durkheim : selected essays. (Book, 1965) [WorldCat.org] [Internet]. Worldcat.org. 2020 [cited 2 November 2020]. Available from: https://www.worldcat.org/title/emile-durkheim-selected-essays/oclc/883981793
- ↑ "Complex number". Britanica. 26 December 2019.
{{cite web}}
: CS1 maint: url-status (link) - ↑ Descartes, René (1954) [1637], La Géométrie | The Geometry of René Descartes with a facsimile of the first edition, Dover Publications, ISBN 978-0-486-60068-0, retrieved 20 April 2011
- ↑ Kline, Morris. A history of mathematical thought. p. 253.
- ↑ W., Weisstein, Eric. "Argand Diagram". mathworld.wolfram.com. Retrieved 19 April 2018.